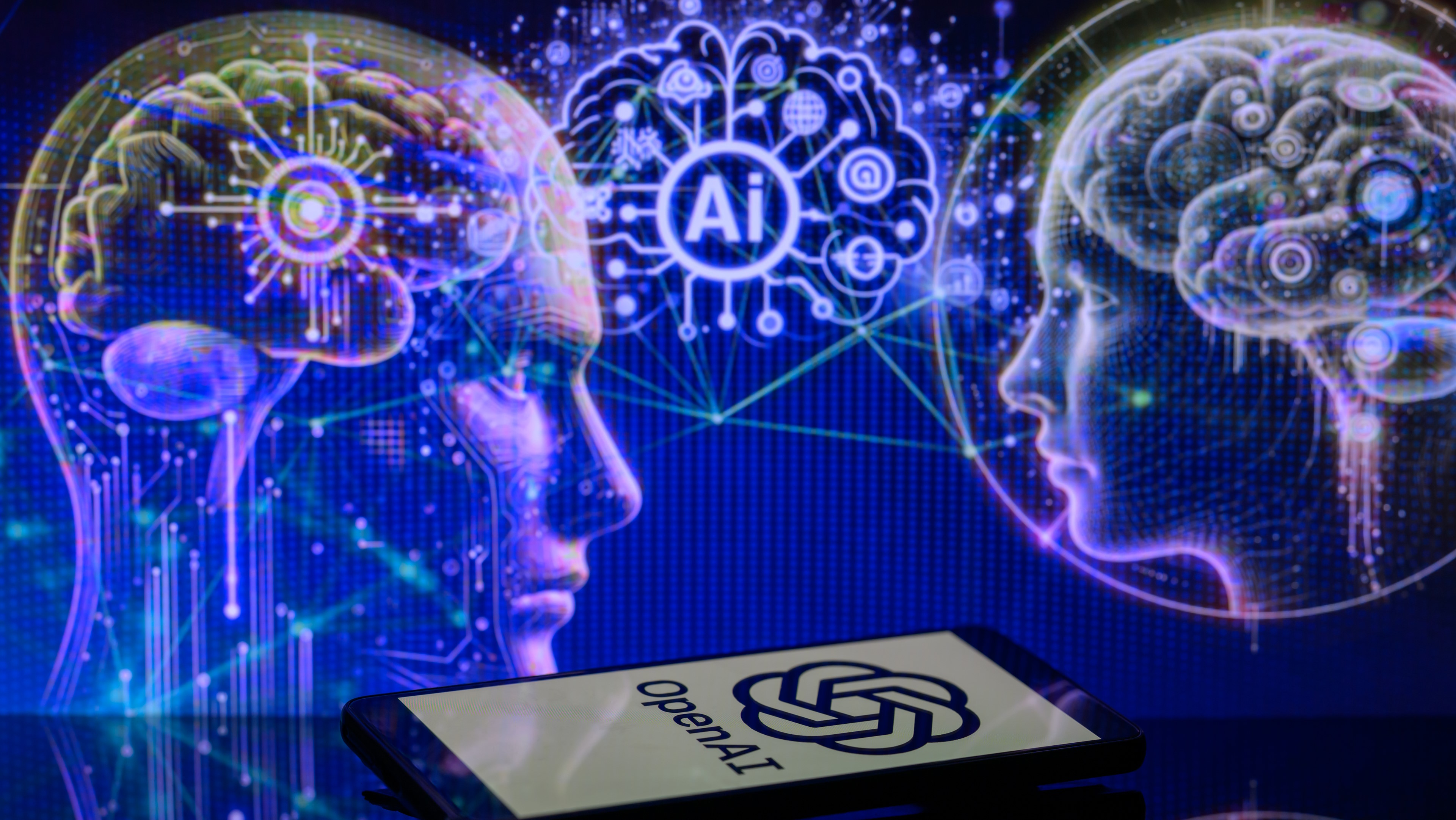
Seems the LLMs have run out of arguments on Reddit to scrape...
Ilya Sutskever, co-founder of OpenAI, thinks existing approaches to scaling up large language models have plateaued. For significant future progress, AI labs will need to train smarter, not just bigger, and LLMs will need to think a little bit longer.
Speaking to Reuters, Sutskever explained that the pre-training phase of scaling up large language models, such as ChatGPT, is reaching its limits. Pre-training is the initial phase that processes huge quantities of uncategorized data to build language patterns and structures within the model.
Until recently, adding scale, in other words increasing the amount of data available for training, was enough to produce a more powerful and capable model. But that’s not the case any longer, instead exactly what you train the model on and how is more important.
“The 2010s were the age of scaling, now we’re back in the age of wonder and discovery once again. Everyone is looking for the next thing,” Sutskever reckons, “scaling the right thing matters more now than ever.”
The backdrop here is the increasingly apparent problems AI labs are having making major advances on models in and around the power and performance of ChatGPT 4.0.
The short version of this narrative is that everyone now has access to the same or at least similar easily accessible training data through various online sources. It’s no longer possible to get an edge simply by throwing more raw data at the problem. So, in very simple terms, training smarter not just bigger is what will now give AI outfits an edge.
Another enabler for LLM performance will be at the other end of the process when the models are fully trained and accessed by users, the stage known as inferencing.
Here, the idea is to use a multi-step approach to solving problems and queries in which the model can feed back into itself, leading to more human-like reasoning and decision-making.
“It turned out that having a bot think for just 20 seconds in a hand of poker got the same performance boost as scaling up the model by 100,000x and training it for 100,000 times longer,” Noam Brown, an OpenAI researcher who worked on the latest o1 LLM says.
Best CPU for gaming: The top chips from Intel and AMD.
Best gaming motherboard: The right boards.
Best graphics card: Your perfect pixel-pusher awaits.
Best SSD for gaming: Get into the game ahead of the rest.
In other words, having bots think longer rather than just spew out the first thing that comes to mind can deliver better results. If the latter proves a productive approach, the AI hardware industry could shift away from massive training clusters towards banks of GPUs focussed on improved inferencing.
Of course, either way, Nvidia is likely to be ready to take everyone’s money. The increase in demand for AI GPUs for inferencing is indeed something Nvidia CEO Jensen Huang recently noted.
“We’ve now discovered a second scaling law, and this is the scaling law at a time of inference. All of these factors have led to the demand for Blackwell [Nvidia’s next-gen GPU architecture] being incredibly high,” Huang said recently.
How long it will take for a generation of cleverer bots to appear thanks to these methods isn’t clear. But the effort will probably show up in Nvidia’s bank balance soon enough.