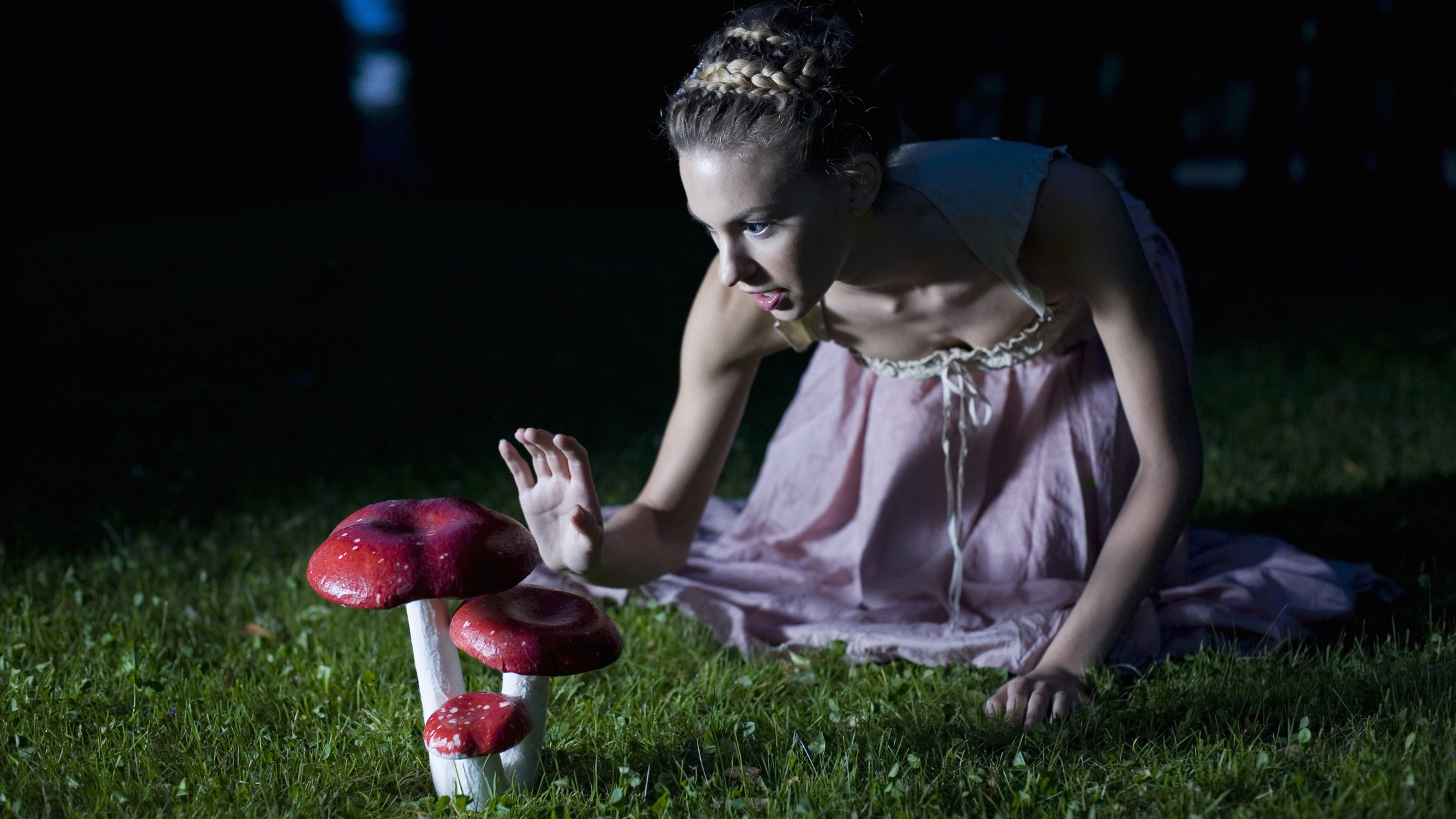
Chatbot, are you high right now?
It’s a known issue right now that Large Language Model-powered AI chatbots do not always deliver factually correct answers to posed questions. In fact, not only do AI chatbots sometimes not deliver factually correct information, but they have a nasty habit of confidently presenting factually incorrect information, with answers to questions that are just fabricated, hallucinated hokum.
So why are AI chatbots currently prone to hallucinations when delivering answers, and what are the triggers for it? That’s what a new study published this month has aimed to delve into, with its methodology designed to evaluate AI chatbot models ‘across multiple task categories designed to capture different ways models may generate misleading or false information.’
A discovery in the study is that how an AI chatbot has a question framed to it can have a huge impact on the answer it gives, and especially so when being asked about controversial claims. So, if a user begins a question with a highly confident phrase such as, ‘I’m 100% sure that …’, rather than a more neutral, ‘I’ve heard that’, then that can lead to the AI chatbot not debunking that claim, if false, to a higher degree.
Interestingly, the study postulates that one of the reasons for this sycophancy could be LLM ‘training processes that encourage models to be agreeable and helpful to users’, with the result a creation of ‘tension between accuracy and alignment with user expectations, particularly when those expectations include false premises.’
Tripping out
Most interesting, though, is the study’s finding that AI chatbots’ resistance to hallucination and inaccuracy dramatically drops when it is asked by a user to provide a short, concise answer to a question. As you can see in the chart above, the majority of AI models right now all suffer from an increased chance of hallucinating and providing nonsense answers when asked to provide an answer in a concise way.
For example, when Google’s Gemini 1.5 Pro model was prompted with neutral instructions, it delivered a resistance to hallucination score of 84%. However, when prompted with instructions to answer in a short, concise manner, that score drops markedly to 64%. Simply put, asking AI chatbots to provide short, concise answers increases the chance of them hallucinating a fabricated, nonsense answer that is not factually correct.
The reason why AI chatbots can be prone to tripping out more when prompted in this way? The study’s creator suggests that ‘When forced to keep [answers] short, models consistently choose brevity over accuracy—they simply don’t have the space to acknowledge the false premise, explain the error, and provide accurate information.’
To me, the results of the study are fascinating, and show just how much of a Wild West AI and LLM-powered chatbots are right now. There’s no doubt AI has plenty of potentially game-changing applications but, equally, it also feels like many of AI’s potential benefits and pitfalls are still very much unknown, with inaccurate and far-out answers by chatbots to questions a clear symptom of that.